Blog
Data Science Vs. Marketing Analytics
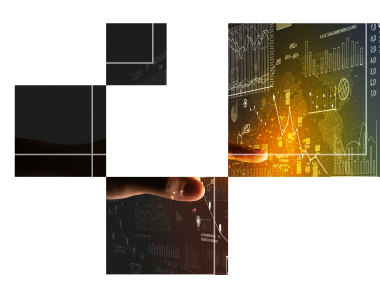
Introduction
In the realm of data-driven modern marketing operations, two commodities that play a vital role are data and analyzing those data. Marketing data are responsible for shaping the domain of marketing analytics. However, this data runs insufficient when it comes to centralizing the database in one place. The insufficiency of data does not meet the demands of marketing analyst practitioners who are wholly dependable on data science for further answering the business questions of organizations. Since these two aspects are closely related to one another, many think that both of them are similar. However, it is quite the opposite. In this article, we will discuss in which ways data science, and marketing analytics differ from one another.
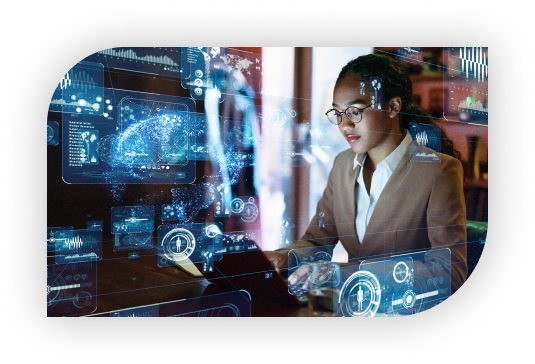
Definition
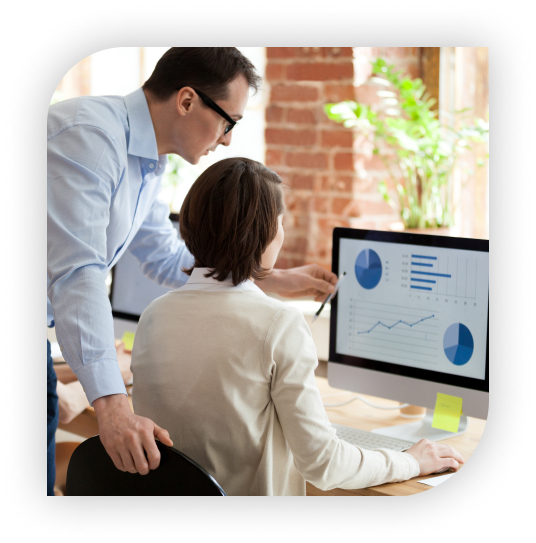
If we understand the overview of both the concepts, differentiating them in deeper senses will become easier. Starting with the background always provides a whole idea and critical highlights of a concept. This section will mention the basic definitions of data science and marketing analytics.
How will one define Data Science?
Data science is a specific field that explores lucrative information from sourced and outsourced data and aids marketers in powerful discerning insights. On analyzing them, any marketer can get insights into customer intent, experience, and preferences that will help them form strategic marketing efforts and derive maximum revenue.
Understanding Marketing Analytics
Marketing analytics is a domain where people study data metrics that enables marketers to determine and evaluate the success rates of the business in a market. This is achieved by measuring product performance, customers' feedback, and critical business metrics such as market attribution, effectiveness, and ROI.
From the definition, we can understand that marketing analytics and data science are the two most important sections of marketing. However, data science mainly includes providing informative data, and marketing analytics aim to evaluate that data and conclude a solution to organizations' business problems.
Future Of Data Analysis Is Now
Data science and marketing analytics are complementary and require closely related skillsets. However, understanding both the domains' objectives and responsibilities will aid in understanding the concepts and their differences at a granular level.
Main Objectives of marketing analytics
- Measuring the outcomes and efficiency of specific marketing strategies.
- Utilizing the data analytics and using it in all marketing campaigns to conduct efficient measures and match the market trends.
- Identifying the effectiveness of online ROI with increasing complexity and variety of marketing channels for positioning a product service.
- Transforming insights and suitable patterns into indicators and strategies for future improvement and product development.
- Having efficient and reliable communication with data scientists and marketing specialists.
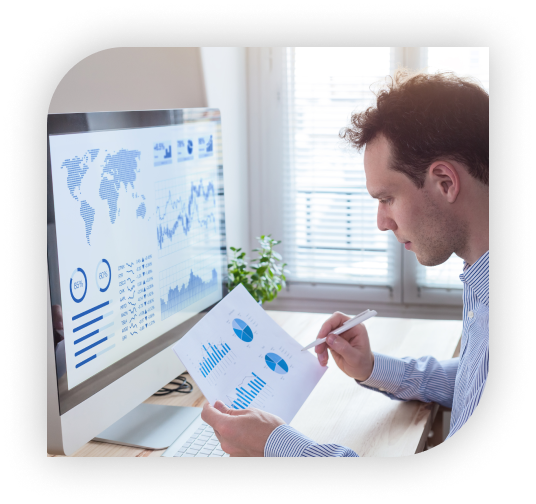
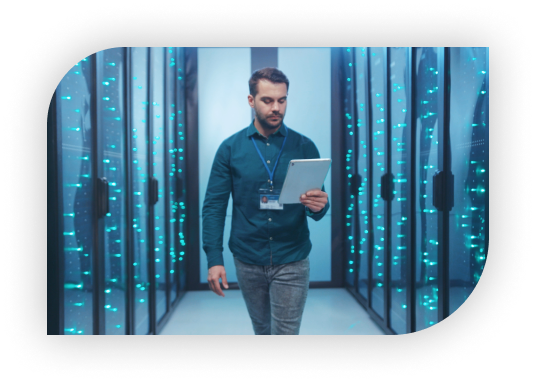
Main Objectives of data scientists
- Applying intelligent algorithms and models to increase the efficiency of marketing efforts for optimization of marketing campaigns.
- Sectioning customers based on coincidences of particular characteristics. This process is called customer segmentation.
- Providing operational data that reflects customers' transactions, interests, and decisions can be an efficient source for real-time marketing analytics.
- Offering lead scoring data for determining the potential customers from the funnel of data. Lead scoring data includes customers’ demographics, behavior, purchasing patterns, web page views, portal visits, likes, shares, and etc.
Critical differences
Since we learned about the domains' primary objectives and what data science and marketing analytics target precisely in its fields, this information will help us in determining the critical differences between them:
- Having significant experience with marketing metrics is essential for marketing analysts, whereas data scientists do not require it.
- Data scientists are expected to formulate evaluative questions for business growth and provide solutions accordingly. On the other hand, the marketing section offers questions to market analysts and asks for efficient solutions.
- Both of the roles require working with the IT team to produce suitable sources. However, marketing analysts are not required to have advanced experience in programming. But the data scientists are required to be professional in programming and creating queries.
- Skills to visualize data and to be able to create a business story out of the sourced and outsourced data must be present in the data scientists. In comparison, marketing analyst practitioners need to focus on marketing metrics.
- The role of data scientists has fluidity and flexibility. They are allowed to work in an accessible form to provide better insights. On the other hand, market analysts work in an inflexible condition where they need to follow a particular direction.
Marketing analytics deal with the practical and technical aspects such as cleaning, sorting, gathering, and studying the data. Market analyst practitioners aid in forming and making improvements in infrastructure, systems, and programs, the operational efforts that read the historical data and diagnostics data. The market analysts are responsible for analyzing past market trends and marketing campaigns.
Whereas data science will offer a clear insight on how the accumulated data will be helpful for the organizations. Data science does not depend on historical data but instead focuses on present data, anticipates recent business trends, and provides data that can predict future trends. Data scientists are more capable of translating the data to actionable business movements. In developing a successful organization in a market, both kinds of expertise are needed to complete the bigger picture.
Conclusion
A data scientist will be doing the math, and the marketing analytic practitioners will be delivering clear reports in a marketing sense. However, there are many times when the data provided is inadequate or wrong. Because of the incorrect data, the analysis made on that will automatically be erroneous. So, making a mistake with the underlying data will ultimately lead to incorrect analysis.
On understanding the provided data, it will open a vast domain of solving common business questions such as:
- How to improve campaign performance?
- What are the best channels to generate quality leads?
- How to transform leads to customers?
- How to transform leads to customers?
Marketers also face some situations where pure data does not help with marketing strategies and theories.